University of Toronto: Researchers use AI to speed reviews of existing evidence
- Global Research Partnerships
- Mar 24
- 2 min read
Updated: 5 days ago
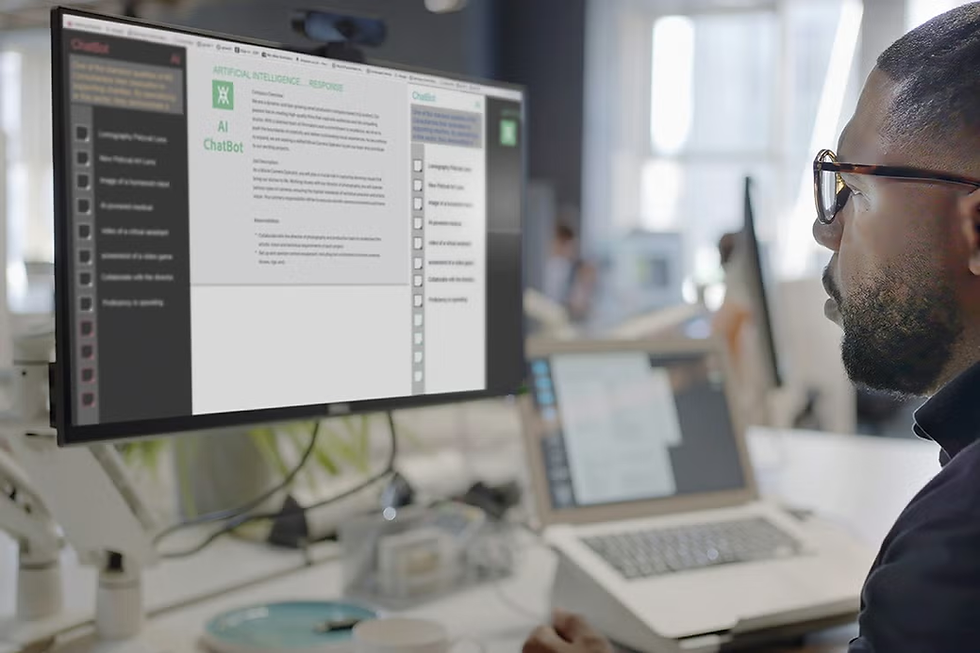
Researchers at the University of Toronto and University of Calgary have developed an innovative approach that uses artificial intelligence to streamline the screening process for systematic reviews, a research gold standard that involves analyzing large volumes of existing evidence.
The study, published recently in the journal Annals of Internal Medicine, involved developing ready-to-use prompt templates that enable researchers working in any field to use large language models (LLMs) such as ChatGPT to sift through thousands of published scientific articles to identify the ones that meet their criteria.
“Whenever clinicians are trying to decide which drug to administer or which treatment might be best, we rely on systematic reviews to inform our decision,” says Christian Cao, the study’s first author and a third-year medical student in U of T’s Temerty Faculty of Medicine.
To produce a high-quality review article, authors first compile all the previously published literature on a given topic. Cao notes that depending on the topic, reviewers filter through as many as hundreds of thousands of papers to determine which studies should be included – a process that is time-consuming and expensive.
“There are no truly effective automation efforts for systematic reviews. That’s where we thought we could make an impact, using these LLMs that have become exceptionally good at text classification,” says Cao, who worked with his mentors Rahul Arora and Niklas Bobrovitz – both of the University of Calgary.
To test the performance of their prompt templates, the researchers created a database of 10 published systematic reviews along with the complete set of citations and list of inclusion and exclusion criteria for each one. After multiple rounds of testing, the researchers developed two key prompting innovations that significantly improved their prompts’ accuracy in identifying the correct studies.
Their first innovation was based on a prompting technique that instructs LLMs to think step-by-step to break down a complex problem. Cao likens it to asking someone to think out loud or walking another person through their thought process. The researchers took it one step further by developing their own approach to provide more structured guidance that asks the LLMs to systematically analyze each inclusion criterion before making an overall assessment on whether a specific paper should be included.